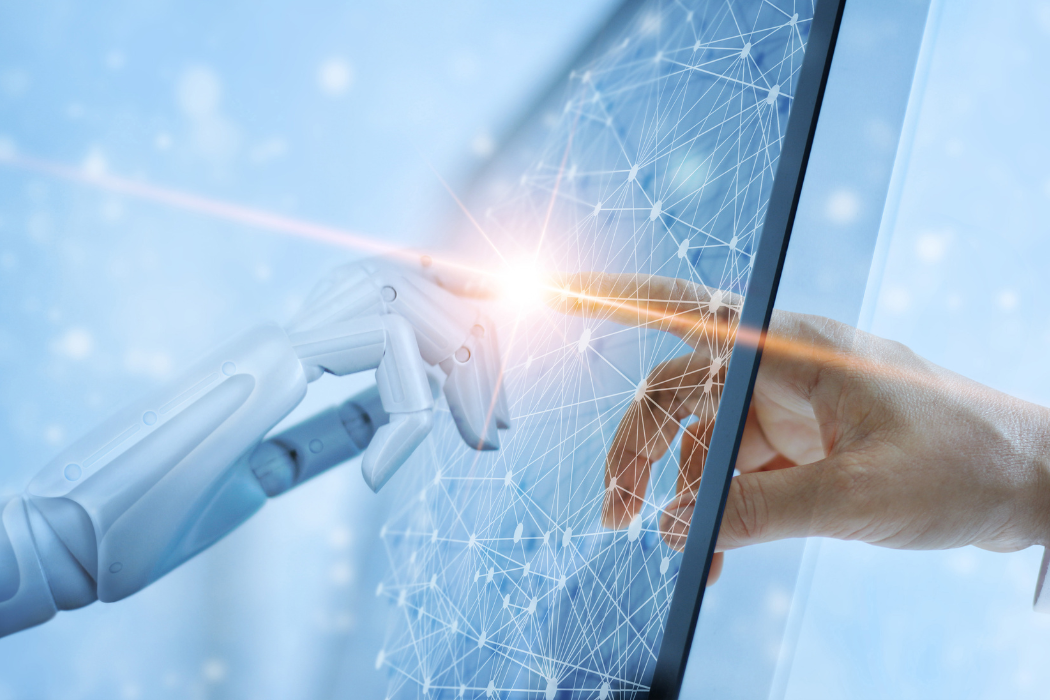
With the explosion of artificial intelligence and rising concerns about energy consumption and associated costs, it’s natural to question how AI advancements can remain financially sustainable. This concern is especially pressing for small and mid-sized businesses wondering if large language models (LLMs) will remain accessible or become exclusive to large corporations.
Only two years ago, the industry faced significant challenges related to processing power and chip availability. NVIDIA, primarily known for its gaming-industry GPUs, found itself in the spotlight as these GPUs became essential for scaling large language models. Pundits predicted that limitations in Moore’s Law and affordability concerns would slow progress, suggesting there was no way to meet demand or to build enough affordable chips to power the AI future.
Just 24 months later, NVIDIA’s market value has skyrocketed from around $300 billion to $3.652 trillion as of November 2024–nearly twice the combined valuation of all 40 DAX companies on the German stock exchange. This unprecedented growth is fueled by substantial investments in AI, the development of next-generation chips, and collaborations with quantum technology firms to incubate new business models. What seemed impossible just two years ago is now reality, underscoring the rapid, exponential pace of technological advancement.
This transformation highlights a key point: technological and scientific breakthroughs often exceed our expectations, leading to solutions that make cutting-edge technologies more accessible and affordable. Just as NVIDIA overcame previous limitations, the AI industry is poised to develop models and systems that will be economically viable for businesses of all sizes.
The Open Question
In the short-term, however, as premium pricing on advanced AI models grows, there is increasing momentum toward open-source alternatives. Open-source models can offer cost-effective solutions, allowing businesses to implement and customize AI without incurring significant expenses. By disrupting the revenue models of major AI companies, open-source approaches could foster a more robust, versatile AI ecosystem. Community-driven improvements could lead to models that are cost-effective, efficient, and adaptable across various industries. However, the risks of open-source AI are evident: once powerful AI tools are freely available, they can be exploited in unintended ways. As highlighted by the release of Meta’s open-source Llama model, open-source AI is already influencing global developments, even in sensitive areas such as military technology. Recent articles even states that China has used Llama to take AI to the battle-ground, questioning the very notion on whether AI could be too powerful to be free.
Brain-Inspired AI Models: Efficiency and Innovation
Another promising path forward is progress. Drawing inspiration from the efficiency of the human brain, AI researchers are developing models with specialized regions for different tasks—architectures that allow quick, energy-efficient responses in some areas, with other areas dedicated to complex problems, much like how the brain operates.
Our brains are remarkably energy-efficient, performing complex computations with minimal energy consumption. The technological replica, by contrast, demands extensive resources. Yet advancements in science and a growing understanding of biology are driving AI models toward higher performance without requiring as much computational power. Over the past 80 years, progress has been exponential. While the Gartner Hype Cycle teaches valuable lessons about disappointments, it also shows how breakthroughs can lead to “quantum leaps” in development. These breakthroughs don’t only lower costs; they open up new possibilities in physics and science as we replicate biological efficiency in technology.
Leading companies like Microsoft with its Co-Pilot and OpenAI’s continuous advancements indicate that in 2025, what I call “Industry AI” will be ready for deployment. These are specialized AI agents with domain-specific knowledge—more streamlined than foundational models yet capable of outperforming them in their respective fields. This marks the most fundamental change in human labor history, as automation and AI agents offer 24/7 capabilities, challenging traditional workforce structures. Smaller businesses will soon have access to AI solutions tailored to their needs without the heavy price tag, thanks to these specialized models designed to reduce computational loads and associated costs.
The Role of Energy Innovations in Reducing AI Costs
Energy consumption is a significant factor in the operational costs of AI models. But advancements in energy technology are set to shift this dynamic. Breakthroughs in fusion energy, alongside a large-scale transition to renewable sources like solar and wind, mean that future energy costs may not be the primary risk. Instead, the focus might shift to competitiveness, with China currently leading in innovations around batteries and renewable transitions.
Moreover, developments in material science are unlocking new opportunities for energy storage and distribution. Improved storage solutions and efficient distribution networks enable energy to be used more effectively, reducing waste and lowering operational costs.
As energy becomes more abundant and affordable, the cost of running high-performance AI models is expected to decrease, making advanced AI solutions accessible to a wider range of businesses, regardless of size.
A New Era of AI Accessibility
While the rising costs of high-performance models present challenges, emerging trends in specialization, open-source alternatives, and innovative business models offer viable solutions. Brain-inspired architectures are making AI models more efficient and less resource-intensive. This convergence of AI innovation and energy efficiency points to a future where AI isn’t just the domain of large corporations but a tool accessible to all, driving growth and innovation across industries.
With the explosion of artificial intelligence and rising concerns about energy consumption and associated costs, it’s natural to question how AI advancements can remain financially sustainable. This concern is especially pressing for small and mid-sized businesses wondering if large language models (LLMs) will remain accessible or become exclusive to large corporations.
Only two years ago, the industry faced significant challenges related to processing power and chip availability. NVIDIA, primarily known for its gaming-industry GPUs, found itself in the spotlight as these GPUs became essential for scaling large language models. Pundits predicted that limitations in Moore’s Law and affordability concerns would slow progress, suggesting there was no way to meet demand or to build enough affordable chips to power the AI future.
Just 24 months later, NVIDIA’s market value has skyrocketed from around $300 billion to $3.652 trillion as of November 2024–nearly twice the combined valuation of all 40 DAX companies on the German stock exchange. This unprecedented growth is fueled by substantial investments in AI, the development of next-generation chips, and collaborations with quantum technology firms to incubate new business models. What seemed impossible just two years ago is now reality, underscoring the rapid, exponential pace of technological advancement.
This transformation highlights a key point: technological and scientific breakthroughs often exceed our expectations, leading to solutions that make cutting-edge technologies more accessible and affordable. Just as NVIDIA overcame previous limitations, the AI industry is poised to develop models and systems that will be economically viable for businesses of all sizes.
The Open Question
In the short-term, however, as premium pricing on advanced AI models grows, there is increasing momentum toward open-source alternatives. Open-source models can offer cost-effective solutions, allowing businesses to implement and customize AI without incurring significant expenses. By disrupting the revenue models of major AI companies, open-source approaches could foster a more robust, versatile AI ecosystem. Community-driven improvements could lead to models that are cost-effective, efficient, and adaptable across various industries. However, the risks of open-source AI are evident: once powerful AI tools are freely available, they can be exploited in unintended ways. As highlighted by the release of Meta’s open-source Llama model, open-source AI is already influencing global developments, even in sensitive areas such as military technology. Recent articles even states that China has used Llama to take AI to the battle-ground, questioning the very notion on whether AI could be too powerful to be free.
Brain-Inspired AI Models: Efficiency and Innovation
Another promising path forward is progress. Drawing inspiration from the efficiency of the human brain, AI researchers are developing models with specialized regions for different tasks—architectures that allow quick, energy-efficient responses in some areas, with other areas dedicated to complex problems, much like how the brain operates.
Our brains are remarkably energy-efficient, performing complex computations with minimal energy consumption. The technological replica, by contrast, demands extensive resources. Yet advancements in science and a growing understanding of biology are driving AI models toward higher performance without requiring as much computational power. Over the past 80 years, progress has been exponential. While the Gartner Hype Cycle teaches valuable lessons about disappointments, it also shows how breakthroughs can lead to “quantum leaps” in development. These breakthroughs don’t only lower costs; they open up new possibilities in physics and science as we replicate biological efficiency in technology.
Leading companies like Microsoft with its Co-Pilot and OpenAI’s continuous advancements indicate that in 2025, what I call “Industry AI” will be ready for deployment. These are specialized AI agents with domain-specific knowledge—more streamlined than foundational models yet capable of outperforming them in their respective fields. This marks the most fundamental change in human labor history, as automation and AI agents offer 24/7 capabilities, challenging traditional workforce structures. Smaller businesses will soon have access to AI solutions tailored to their needs without the heavy price tag, thanks to these specialized models designed to reduce computational loads and associated costs.
The Role of Energy Innovations in Reducing AI Costs
Energy consumption is a significant factor in the operational costs of AI models. But advancements in energy technology are set to shift this dynamic. Breakthroughs in fusion energy, alongside a large-scale transition to renewable sources like solar and wind, mean that future energy costs may not be the primary risk. Instead, the focus might shift to competitiveness, with China currently leading in innovations around batteries and renewable transitions.
Moreover, developments in material science are unlocking new opportunities for energy storage and distribution. Improved storage solutions and efficient distribution networks enable energy to be used more effectively, reducing waste and lowering operational costs.
As energy becomes more abundant and affordable, the cost of running high-performance AI models is expected to decrease, making advanced AI solutions accessible to a wider range of businesses, regardless of size.
A New Era of AI Accessibility
While the rising costs of high-performance models present challenges, emerging trends in specialization, open-source alternatives, and innovative business models offer viable solutions. Brain-inspired architectures are making AI models more efficient and less resource-intensive. This convergence of AI innovation and energy efficiency points to a future where AI isn’t just the domain of large corporations but a tool accessible to all, driving growth and innovation across industries.
So perhaps the greater concern today shouldn’t be whether small and mid-sized businesses can afford AI, but whether–in an increasingly winner-takes-all market dominated by tech giants– there will be any small-/mid-sized businesses left to worry about.So perhaps the greater concern today shouldn’t be whether small and mid-sized businesses can afford AI, but whether–in an increasingly winner-takes-all market dominated by tech giants– there will be any small-/mid-sized businesses left to worry about.